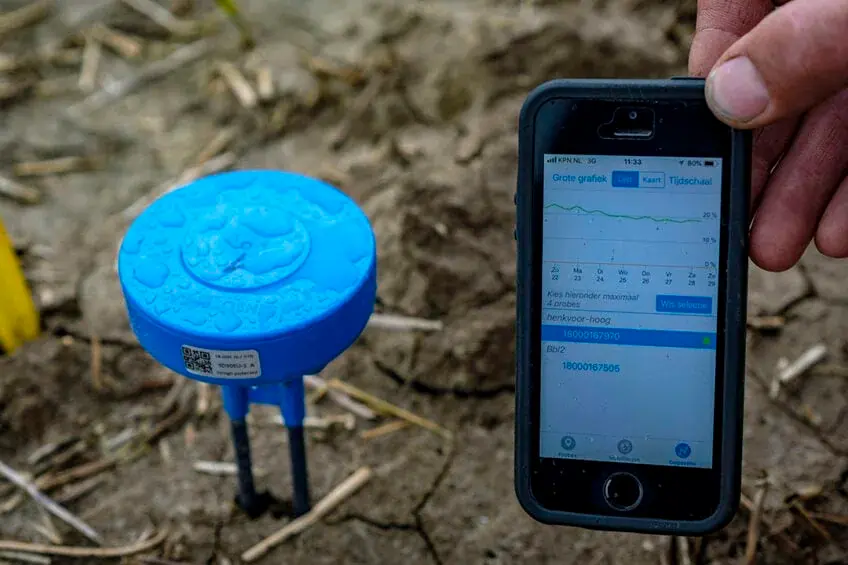
The new book ‘Advances in sensor technology for sustainable crop production’ brings together the knowledge of the world’s top researchers in the field of sensor technology. We asked one of the authors Dr Ken Sudduth, a Research Leader at the US Department of Agriculture, six pressing questions.
Which knowledge shocked you the most? And what was the biggest revelation?
“The editors have done a commendable job bringing this book to fruition. I think the most important part of the book to me was the section on advances in sensor data analytics. As we move forward in this field, the combining of multiple sensors to address a need (sensor fusion, Chapter 10) along application of artificial intelligence analysis techniques such as machine learning (Chapters 8 & 9) in turning sensor data into actionable information will be vitally important.”
What are the three biggest (potential) drawbacks of the increasing use of sensors in agriculture and specifically for farmers?
“My response is more in terms of cautions to be aware of when using sensors. One caution is to regularly check the accuracy of the sensor system. For example, the need to check and re-calibrate the usual impact-based combine yield monitor is well documented. Calibration needs to be checked for each crop and harvesting condition encountered (such as high-moisture grain vs. standard moisture) and at least annually. However, a large number of farmers don’t take the time to follow this process, which can dramatically increase the error in their yield measurements. This same caution holds for many soil and crop sensors as well.”
One caution is to regularly check the accuracy of the sensor system
“A second caution is to understand what the sensor is actually measuring. This can be as simple as understanding the volume of soil that affects the measurement provided by a soil moisture sensor. If the goal is to know moisture in the seed zone at planting, but the sensor gives an integrated reading over the top 20 cm of soil, that measurement may not be very useful for the intended task.
A third, related, caution relates to using more complex sensing systems to make indirect measurements. For example, a soil sensing system might include multiple sensors that are measuring different aspects of the soil environment, but not directly measuring the variables we’d like to use for making agronomic decisions. In that case, a number of “ground truth” measurements may be used with advanced modeling, like machine learning, to relate the output of the multiple sensors to the useful soil variables. Because the measurement is indirect, it’s accuracy is dependent on the data used to develop the model. You might have highly accurate estimates when soils are similar to those from which the ground truth measurements were acquired. But if you take the same sensing system to an area with quite different soils, the estimates may not be accurate.”
In 1994, the first time a GPS yield monitor on a combine was used to record grain yield site specifically in the USA. It gave precision farming the kickstart. Which three sensors do you think have had the biggest impact in agriculture to date?
“Certainly, the grain yield monitor/sensor must be one of the three. Yield translates directly to income for the farmer, making it the data that’s of most immediate value. Another would be the various sensors that are available to measure soil electrical conductivity (or resistivity, Chapter 6). Conductivity sensing is widely practiced worldwide and gives farmers and researchers a quick measure of soil variability. This can be used for simply visualizing spatial soil differences or can be calibrated to important soil properties such as texture. The third sensor (or sensing approach) I’d mention is remote sensing in the visible and near-infrared (VNIR) wavelength regions. This type of sensor has facilitated within-season monitoring of crop health and vigor, usually through the calibration and mapping of vegetative indices. Maps of these VNIR-based vegetative indices enable directed crop and pest scouting and can also provide a data layer for within-season application of crop nutrients.”
Which three new type of sensors will have the greatest impact on crop production in the (near) future and why?
“Water availability and use is becoming more and more important for sustainable agriculture. Therefore, sensors that can provide data for efficient irrigation management will have a large impact, particularly when coupled with irrigation systems that can vary water application spatially as well as temporally. Soil measurements would include water content sensors and electrical conductivity sensors that can be used to develop the soil texture maps needed to help interpret soil water content data. Remote sensing of crop water status through the growing season would also be needed to trigger an irrigation decision support system.”
Water availability and use is becoming more and more important for sustainable agriculture
“Similarly, efficient crop nutrient management, especially of nitrogen, is important for profitability and sustainability. Detection of plant nutrient stress through proximal or remote optical sensing is possible; however, nutrient application schemes based only on these data are not always successful, as they don’t provide an indication of growing conditions through the remainder of the season. Coupling crop sensors with sensing of soil nutrients, long-term weather data, and simulation models may be a promising way forward for in-season nutrient application.”
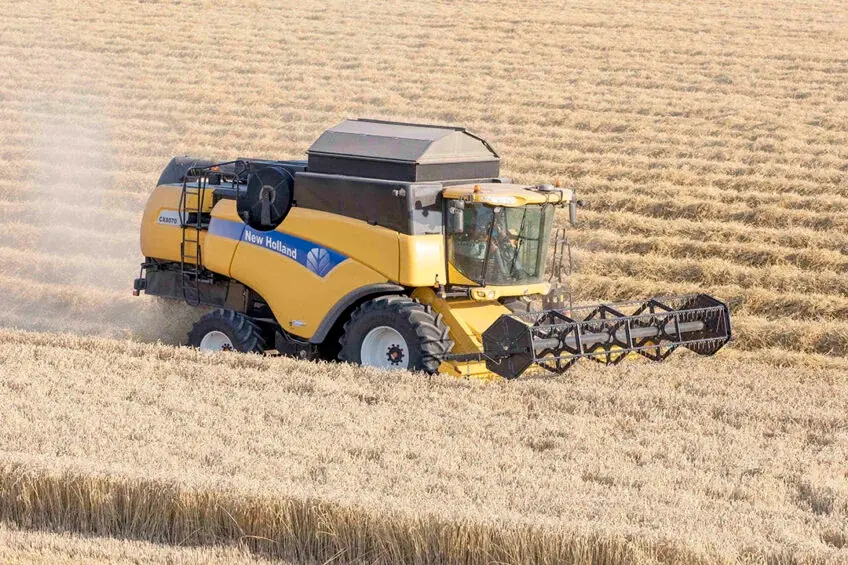
There are also technological developments that counteract the reliability and usability of data coming from sensors. For example, the increasing working width of combines with cutting bars up to 18 meters wide ( 60 feet) which makes yield observations less accurate. What can be done about this trend?
“That’s a good example, but to my way of thinking, the increasing width of combines just means that yield observations are less spatially dense. Because of more flow being averaged into each measurement, that measurement may actually be a more accurate representation of the harvested crop yield, however over a larger area. Whether the less dense measurements are a problem or not probably depends on the intended use. If yield data are being used to, for example, guide fertilizer replacement based on crop removal, this scale may work well. At least in my area, the standard fertilizer applicator is 60-70 ft wide and has no provision for varying rates across the boom. That being said, I think I’ve seen some sensor fusion work where measurements of biomass variations across the working width were being combined with the grain flow data to estimate yields at a narrower width.
Another technological development that might affect sensor accuracy is the fast turnover in commercial crop hybrids and varieties. A crop sensing system may be developed using a group of varieties in common use at the time as “ground truth”. However, if that sensor comes to market a few years later, those same varieties may no longer be in common use. Performance of the sensor might or might not be similar with newer varieties; this would need to be determined.”
So there are more and more sensors collecting tons of data in the soil, air and crops. How should a farmer process all that data and, above all, extract useful information from it?
“Yes, that is certainly a key point. Many farmers will tell you that they are awash in a sea of data and need help. They need new tools and processes to help them turn data into actionable information and then into good management decisions. In my opinion, this needs to be a focus for those of us involved in research and development of ag sensor technology. There are several key areas where improvements are needed.
First, we need to make data collection and aggregation easier. There are multiple parts to this process: easier, more robust sensor setup and calibration, improved data transfer from the sensor to the point of analysis, and standardization of data formats across sensor types and manufacturers. The point of this first step is to streamline the process of collecting data and getting it processed for analysis, recognizing that we often need to combine multiple data streams for best results.”
We need to provide farmers with reliable and accurate analysis models
“Second, we need to provide farmers with reliable and accurate analysis models that can turn this processed data into information. Model development is only part of the need here; another part is to define under what conditions the model reasonably applies (geographic area, soil type, etc.) so that it isn’t used to make inaccurate inferences.
Third, we need to present the information to the farmer or other decision-maker in a way that is understandable to them and allows them to examine “what-if” scenarios. We should recognize the importance of providing farmers with the tools needed to support their decision-making, rather than merely presenting them with an already-made decision.
This task of developing and deploying systems that help farmers turn data into information into decisions is complex and critically important. It requires partnering of researchers, agronomy and equipment companies, data services, and of course the farmers themselves to achieve the desired result.”
Commentaires